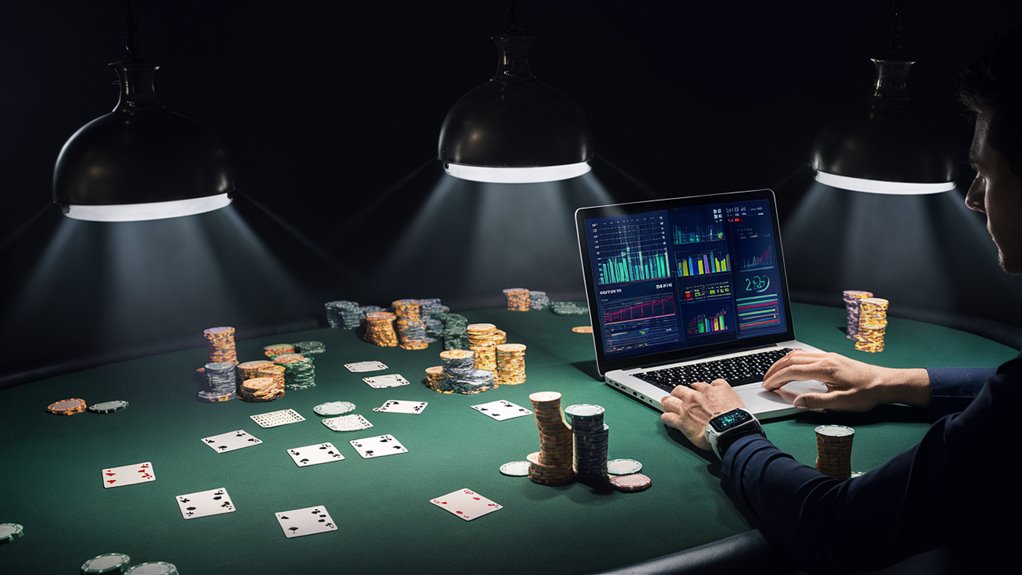
How Gamblers Can Use Data Analytics to Their Advantage
How Gamblers Can Use Data Analysis to Benefit Themselves
The introduction of data analysis has changed the way a successful gambler approaches their choices and strategies. By examining raw figures carefully, punters transform their information into ideas with power, and as a result, derive cash flow that is much more rewarding for them. Its systematics comprise such varied techniques as: examine the random information values regularly, compare records of people, contrast games in logical groups, or consider all even numbers and their corresponding consecutive odd numbers.
Statistical Analysis and Pattern Recognition
Advanced statistical methods turn historical gambling data into tools of prediction. By following through on data acquisition procedures, gamblers are able to keep track of key variables such as:
- Historical performance metrics
- Movements of the market
- Environmental factors which may affect a game’s outcome
- Trends in odds fluctuations
Predictive Modeling Applications
Gamblers are able to use machine learning algorithms and regression analysis tools in order to:
- Find any inefficiencies that exist within the betting markets
- Determine where value opportunities lie
- Pinpoint the chances of particular outcomes
- Work out in advance how individual performances will go
Risk Management Optimization
By applying portfolio theory methods, gamblers can:
- Spread risk around whilst betting
- Determine the most profitable size of investment
- Effectively manage their bankroll
- Keep an eye out for where risks pay off handsomely compared with
Data-Driven Decision Frameworks
Gambling strategies based on data analysis require the following:
- Continuous operational monitoring
- Real-time data monitoring
- Dynamic strategic adjustments
- Comprehensive records
Using these advanced analytical techniques, gamblers can develop complex betting systems that will give long-term benefits over a broad range of gambling markets.
The Rise of Data-Driven Gambling
The Evolution of Data-Driven Sports Betting
Applying advanced analytics in today’s betting:
With an eye towards data analytics and machine learning technologies, the landscape of sports betting has been dramatically changed. Sophisticated algorithms now process extensive historical data sets, converting raw statistics into valuable betting intelligence. Professional gamblers are increasingly using database-driven methods for quantitative analysis to reveal patterns in the betting markets which are not visible to others.
Real-Time Data Integration and Statistical Analysis
Today’s betting platforms are now equipped with quantitative data streams which give punters live information on key variables. By means of these systems, they are able to monitor performance metrics more fully, including such things as:
- Team statistics and historical performance
- Head-to-head competition records
- Player injury status and team roster changes
- Environmental factors, venue conditions
- Social media sentiment indicators
Advanced Predictive Modeling Technologies: Sportsbook Software
The use of predictive analytics represents a Roulette Revolution significant technological improvement over traditional sports betting methods. Its statistical modelling techniques include:
- Regression analysis
- Neural networks
- Machine learning algorithms
- Pattern recognition systems
Through these tools, the future holds a great promise for accuracy in predicting game results and finding winnable betting opportunities.
The Integration of Technology and Expert Analysis
Automation offers an exacting mathematical foundation, but achieving a winning betting strategy depends on combining machines with human expertise. This method of approach combines:
- Quantitative data analysis
- Market psychology analysis for situational context
- Evaluation of historical trend interpretation
The integration of computational muscle with human knowledge really does represent the apogee of today’s data-driven gambling methodology.
Statistical Analysis in Gambling
Understanding Statistical Analysis in Gambling through A Beginner’s Guide
Key statistical concepts for successful gambling:
Statistical analysis is the basic framework upon which any plan for betting must be based. Probability distributions, variance calculations, and expected value analysis furnish data-driven decision-making. By systematically dissecting historical betting patterns and translating them into more scientific forms such as advanced statistical models, one is able to develop betting strategies that are robust and overcome traditional ones.
Gambling Statistics
The three pillars of thoughtful analysis in successful wagering are: probability evaluation, risk analysis, and outcome prediction. Standard deviation gives key insights into betting volatility, while regression analysis opens up important patterns contained within historical data. Understanding the law of large numbers and central limit theorem enables bettors to make mathematically sound decisions.
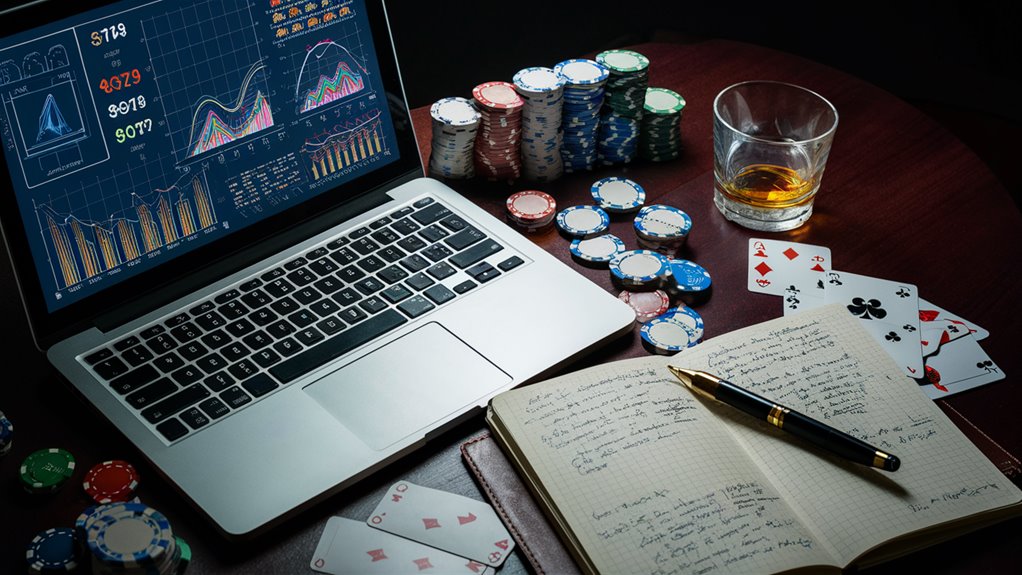
Advanced Statistical Tools and Using Them
After you’ve got the basics of probability calculations down, a great number of analytical possibilities open up to try for size. By using Bayesian analysis, you will be able to refresh the odds at any time as fresh data comes in. Monte Carlo simulations allow intelligent, comprehensive models of results to be delivered.
By fusing these statistical methodologies with professional stake management, your gambling strategy will no longer be based on groundless reasoning. If you grasp these advanced concepts, you will see that an organized, data-driven framework will determine every period for which to act in profit-taking and decision-making at the tables.
Tools of the Trade
Developing an Efficient Data Infrastructure
For a complete system of data collection, strategic use of the localized systems. The founding keystone for effective data gathering operations includes high-end tracking software, automatically collected data channels, and enterprise databases.
Basic Components of Data Collection
Utility tools on the real-time analytics platforms:
Efficient analytics platforms provide systematic collection facilities for important factors and indicators. Standard applications like advanced data transmission systems monitor key performance indicators, environmental parameters, and market movements. These tools all help to carry out comprehensive documentation of pattern variation, statistical characteristics, and Pinnacle Portal performance indicators.
Data Storage Methods
A company’s database infrastructure is used to store historical data. While structured file management systems such as SQL enable data control, storage is still essential for cloud solutions to ensure reliability and accessibility. By creating automatic backup steps and certifying the data entered, data integrity and accuracy are preserved.
Integration and Everything Else
Connecting to additional public datasets also offers these tools, such as full performance statistics and market analytics available at the API level. User-specified analysis models plus a mix of thematic software tools make a system to analyze data as a whole. This infrastructure provides evidence-based decision-making through integrating data collection with a systematic analysis process.
Advanced Data Management Features
- Real-time data capture and processing capabilities
- Automated tracking of key performance indicators
- Cloud-based storage using application
- Statistical analysis tools for comprehensive evaluation
- API tying-in for extended data access
- Automated back-up and checking routines set in place
This powerful system guarantees the efficient collection, storage, and analysis of vital data items with integrity and versatility.
Recognizing Patterns and Trends
In Data Analytics, Recognizing Patterns and Trends
Observing statistical patterns and trends:
Identifying patterns and trends is an indispensable part of modern data analytic strategies. Recognizing patterns requires systematic observation of repetition in similar events, sequences, and interrelations among vast complex data sets. Knowing both cyclical patterns and linear trends through historical data analysis is the foundation of effective prophetic modeling.
Advanced Analytical Tools and Methodologies
Professional data analysis software will uncover statistical anomalies and lay down standard patterns spanning diverse scenarios. Key metrics include win-loss ratio monitoring, volume analysis, and real-time price movement. On the other hand, when complex data sets are broken down into small segments, possible emerging patterns quickly reveal themselves.
Multi-Variable Analysis and Prediction Models
Integration of time series analysis, probability distributions, and regression modeling provides a comprehensive basis for analysis. With a purely statistical approach, the prime factors to look for are seasonal variations, market sentiment indicators, and behavioral pattern analysis. A new generation of advanced predictive simulations takes all these into account in its search for future trends, with an eye on fine pattern distinctions and strict validation through continuous methodological refinement. 온카스터디
Key Components of Pattern Analysis
- Validation of statistical trends in time
- Pattern identification techniques
- Model development for forecasting
- Time series correlation analyses
Risk Management Through Analytics
Risk Management Through Analytics: Advanced Data-Driven Strategies
Risk management through analytics has become a standard part of modern decision-making frameworks. By employing sophisticated analytical tools and data-driven methodologies, organizations can systematically assess potential risks and strategically optimize outcomes. Normal statistical characteristics and probability distribution analysis help to define the potential loss. Quantitative limits for risk tolerance can be established.
Key Performance Metrics and Risk Evaluation
Advanced risk metrics are essential for maintaining operational control. The Sharpe index serves as a crucial indicator of performance which has taken into account risk, while Value at Risk (VaR) measures give clear estimates on how much you can expect to lose within certain confidence intervals.
Some reliable measures in this paper are breaking down long-standing assumptions, the design of decision-making frameworks, and sustainable range management systems. Through segment dynamism and risk division, correlation analysis between market segments becomes effective strategies of risk diversification.
Real-Time Analytics and Risk Management
Real-time analytics enable quick identification of moving market conditions, allowing for strategic maneuvering that reduces risk and catches opportunities with high returns. The comprehensive system ensures that the risk-reward ratio is in the investor’s favor all of the time. Basic components of analytics include:
- Statistical modeling tools
- Risk management frameworks
- Performance tracking systems
- Market correlation indicators
- Real-time monitoring solutions
Predictive Modeling in Sports Betting
Advanced Predictive Modeling in Sports Betting: A Comprehensive Guide
Data-driven forecasting fundamentals:
The predictive modeling attached to sports gambling is now a sophisticated, big data-based process, melding large-scale statistical analyses and advanced machine learning algorithms. Modern forecasts are built on massive databases that gather and organize team performance indexes, individual player statistics, historical matches, and often ignored factors that could greatly affect outcomes such as weather or time of year. This needed, in turn, allows us to write proprietary software that does chain reaction analytics data needed in real-time.
Market Efficiency and Model Optimization
Successful betting models identify and exploit market inefficiencies at the intersection of predicted values versus bookmakers’ odds. Essential elements include:
- Statistical tests to ensure that your model continuously performs better than chance
- Ensuring up-to-the-minute recalibration of your models
- Bayesian recalibrations to keep your systems up-to-date with new data
For validation purposes, separate training and testing datasets are employed in the model.